How industries could benefit from real-time data analysis and event prediction
Data is gold, don’t miss out on it
Here’s an example.
Let’s say a typical software is like a thermostat. It operates in a simple loop, always returning the room to the set temperature. It might work and keep the temperature steady, but it doesn’t learn.
By contrast, a smart thermostat will learn from data and become much more efficient over time. The smart thermostat would test each idea and repeat the process. We call these types of systems interoperable because they can exchange and make use of data.
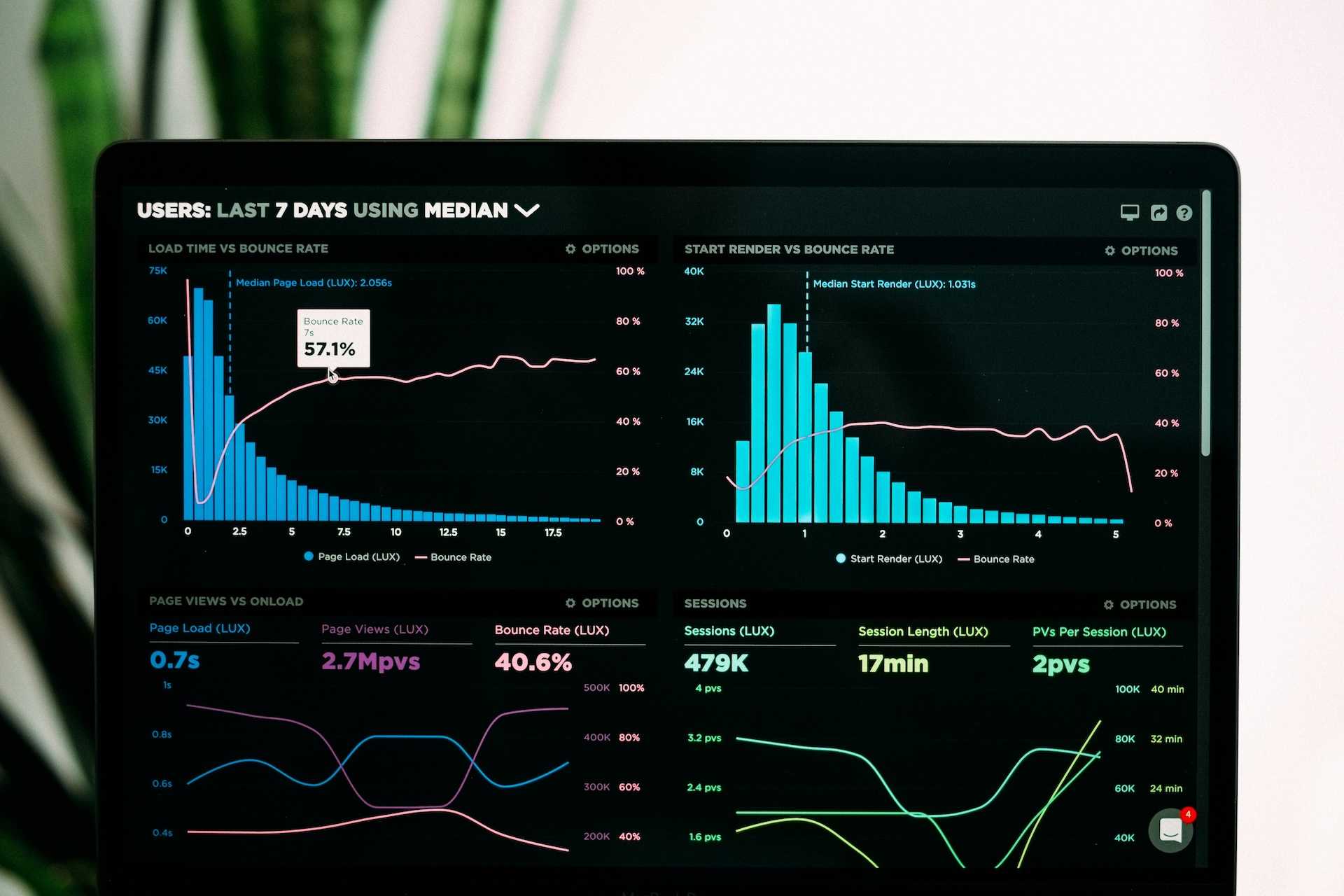
Photo by Luke Chesser on Unsplash
And this is how real-time data analysis works when it’s properly integrated with your business operations.
Why is this important? Each extra moment that delays an improved decision also raises the cost of your operation. By using real-time analytics, problems get solved faster but not only that, decisions are made based on real data.
/Real-time analytics is the discipline that applies logic and mathematics to data to provide insights for making better decisions quickly./
Downsides of outdated data analytics
There is still a perception out there that custom made solutions come with a great cost — but they should be seen as an investment. We’ve already talked about the benefits that come with tailored solutions where we went through some of the main competitive advantages.
Here, we’re going to look at the specific costs that may come with outdated analytics and reporting.
The first one is pretty straightforward, it’s the quantifiable cost of having to collect, store and analyze data. With outdated systems, your data will not be used properly and missed opportunities will cost you time and, in most cases, money.
Secondly, there is the cost of collecting, storing and using “old” data. The more data you have, the faster it changes (in some cases, this can be seconds) and gets old. When you analyze old or outdated data, your analysis is going to be outdated or even worse, skewed, making it useless for future use. Maintaining data accuracy and keeping it up-to-date should be a priority when you use data to inform your future business decisions.
Then there is timing. Being agile, proactive and having the ability to react immediately is of the utmost importance to stay competitive and at the top of your game. Businesses that are faster tend to do better than those that get lost in decisions. Real-time analytics makes it possible to spot issues as they arise so you can address them before they grow or affect more aspects of the business. Real-time analytics also enable your team to deal with end-users or customers more quickly, which means more satisfied customers.
Solutions like Tray can help you overcome these challenges.
No more errors, redundant data, or slow data processing. With Tray, your business will become more stable, faster and better at data automation than ever before.
Meet Tray, our own real-time analysis solution
Tray applies advanced incremental machine learning on data streams to effectively and easily predict the load of external data sources.
The biggest challenge in the software design of interoperable systems for sustainable data exchange is how to handle unpredictable availability, (un)reliability and (non)responsiveness of the integrated external data sources. By integrating the innovation Tray, a dynamic throttler of throughput, into an interoperable system, such problems can be effectively solved.
Who can benefit from Tray?
This Medius innovation was made to be implemented across all forward-thinking industries like fintech, insurance, pharma, energetics, and all those businesses that operate through many different applications or/and software.
We know that each organization uses many different software solutions, from custom to off-the-shelf, sometimes outdated and without support. This is something we saw as an opportunity and that’s why Tray is technologically agnostic, which means it has no limits and can be implemented over any system while not interfering with the process.
When every system operates by its own set of rules, when you don’t have a common ground, you need a tool that makes solutions from different origins work together.
How do we know all this and how can we be sure Tray will work for you? Well we’ve tested it — in real life and on a large scale (500,000+ users) — and it works like magic.
Let’s look at Tray in action
The basic purpose of interoperable systems is to enable the customer to transparently, quickly and reliably query data from various data sources.
And this is exactly what we do with Tray, which was first used and implemented within a complicated government system, where we identified the opportunity and offered them the implementation within their interoperable information system for uniform execution of smart data queries.
That interoperable system was originally built to obtain data for one source, but as a multi-purpose common application building block, it has become widely used by many other government systems.
Among other things, it supports the acquisition of data on the property and income status of social rights applicants and their family members, mostly from data sources within the public administration, but also from external sources. In short, it’s a big system with a lot of data that needs to be processed in real-time.
So what’s the challenge?
The system conducts tens of thousands of inspections each day. As mentioned already, the biggest challenge for architects and developers of interoperable systems is the unpredictable availability, (un)reliability and (un)responsiveness of many data sources.
Developers of such systems often resort to performance optimization by introducing the so-called “queues” where the system accumulates requests that have not yet received responses. These queues are assigned self-replicating actions to ensure that resource queries are performed again. This allows developers to obtain a delayed response from newly available sources that were previously unavailable or responded with an error.
The crucial question here is how to optimally empty and fill the queues at the same time. If the queue is emptied too quickly, there is a high probability that the external data source will be overloaded again and as a result, the entire system will be congested. However, if on the other side, all requests are queued, responses will be delayed, which does not reflect the optimal performance of the system. This is where Tray comes into play and saves the day.
Tray offers an answer to the question of how fast to empty and fill requests to achieve optimal throughput of an interoperable information system. The aim is the smooth operation of the system and the consequent rapid acquisition of data (in this case) on the property and income status of applicants for social rights.
Tray optimizes the performance of an existing interoperable system using advanced machine learning elements. Tray includes open interfaces that build a model based on past metrics to predict the throughput or load of an individual data source in real-time. These predictive models are calculated using various machine learning methods and algorithms. The damping component of the interoperable system thus obtains dynamic parameters for charging and discharging the memory types of requests in the interoperable system by calling the open REST API of the microservice.
Each time Tray incrementally corrects predictive models, it calculates the characteristics of new state patterns of an interoperable system. Thus, predictive models also take into account possible updates of external data sources, which are measured by metric data in the context of calls from an interoperable system.
Before and after Tray
The currently described Tray system is used in production operations as a support component to the complex interoperable information system, where it enables more robust, stable operation of government operations even in extreme situations when there is a flood of input requests. Before the installation of the innovative Tray solution, a large number of input requests resulted in data source failures. As we are talking about extremely large amounts of requests for verification (tens of thousands per day on average), outages in the system occurred frequently, which limited the up-to-date treatment of applicants and caused many problems.
Tray’s success is reflected in fewer outages of external data sources and also in the faster average time required by the system to respond to users.
As a result, applications from social rights applicants at social work centers are being processed more quickly, and the user experience has also significantly improved.
Not only a story of success but a real win-win situation!
Data is gold, don’t miss out on it!
Let’s end with this. We live in times where data is gold and every missed opportunity can cost us time or money — or both! With real-time analysis, data gets used properly and, as a consequence, your system continues to learn, grow and become stronger which gives you more opportunities to connect with users at the right moment, with exactly what they need from you.
And this is how long-term business is conducted.