Why Is Machine Learning Relevant Now?
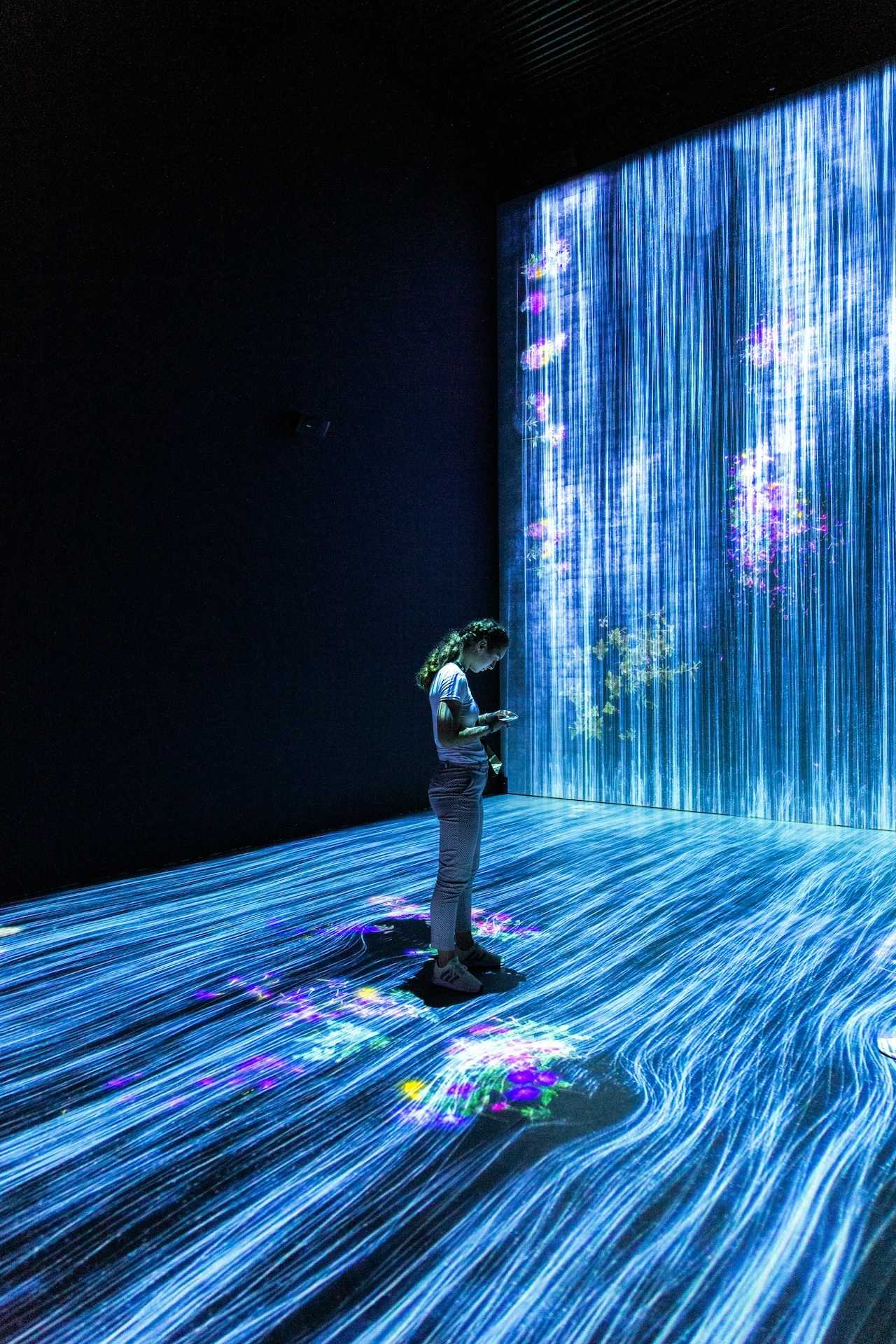
Photo by mahdis mousavi on Unsplash
With a formidable role in driving the efficiency of analytics, machine learning is transforming many markets, including traditional ones, such as Insurance, Banking, Government, Manufacturing, Pharma, etc.
While machine learning exhibits promising potential for the future, it is important to identify the market opportunity for machine learning.
Does it have any scope for growth? Let’s look at the numbers:
Almost 50% of respondents in a study by McKinsey in 2020 affirmed the adoption of AI in at least one business function.
According to a report by Wall Street Journal, the improvements in AI and machine learning could drive GDP growth by 14% by 2030.
Organizations can experience around 54% improvement in productivity through the use of AI.
On top of it, around 91.5% of top businesses worldwide currently have active projects in AI and machine learning.
Applications of Machine Learning
With machine learning, computer systems can take all the customer data and utilize it It operates on what’s been programmed while also adjusting to new conditions or changes Algorithms adapt to data, developing behaviors that were not programmed in advance.
Deep learning is a segment of machine learning In essence, it’s an artificial neural network with three or more layers Neural networks with only one layer can make estimated predictions The addition of more layers can assist with increasing optimization and accuracy.
Here are two examples of how machine learning can be applied to improve the business processes in insurance and telecommunications:
Insurance
The untapped source of data
Most insurance companies are capable of processing around 10% of the data they can access. A major share of the data insurance companies could access today rests largely in structured data in traditional databases As a result, insurance companies fail in unlocking value from structured data while also overlooking the value hidden in unstructured data The advantages of machine learning with new data analytics technologies could help insurance companies derive valuable business insights for all processes throughout the insurance value chain Some of the notable applications of machine learning and AI in insurance include expense management, fraud identification, assessment of premium leakage, risk appetite, and litigation management.
One of the prominent examples of practical use cases of machine learning in insurance includes real-time insurance advice Different algorithm methods (i.e supervised learning, unsupervised learning, and reinforcement learning) can offer personalized solutions where consumers could customize profiles and receive recommendations for products that fetch the best value In addition, the use of chatbots and messaging apps is driven by machine learning to establish more value for the insurance sector beyond the prediction on different products Another potential use case of machine learning in the insurance sector is evident in faster claims processing.
Telecommunications
Find the anomaly
Machine learning algorithms have also shown exceptional value benefits for the telecommunications sector The prominent use cases of machine learning in the telecom sector include anomaly detection, network optimization, root cause analysis, and managed services.
Take note:
Years of experience in the telecom sector have given Medius a clear overview of the practical use cases of machine learning in this sector, with anomaly detection being the most prominent one.
Machine learning can help drive system monitoring efficiency by significantly improving anomaly detection systems in telecom networks The anomaly detection systems can then help proactively identify performance issues alongside discrepancies in network behavior With the proactive discovery of anomalies in KPIs, telecom networks can round upon the root cause of problems before they even happen. The real-time applications of machine learning in telecom also include effective data analysis for trouble ticket management Machine learning algorithms can provide effective classification, prioritization, and escalation of incidents At the same time, insightful churn prediction also facilitates improved customer retention and capacity planning Furthermore, the use of Big Data supported with new technologies like reinforcement learning and deep learning can drive the automation of network design processes You should also notice the value of machine learning for ensuring real-time optimization of network performance in telecommunications.
We can all agree that machine learning is a remarkable technology in the field of artificial intelligence Even in its earliest applications, machine learning has already improved our daily lives and transformed our vision of the future On the other hand, the world should look for more innovative ways to use machine learning. As of now, machine learning is transforming many significant elements of real-world infrastructure such as the Energy sector, from power grids to Oil&Gas, supply chain management systems, etc However, machine learning applications are not limited to the above-mentioned markets and industries Some of the newest use cases and success stories come from the fintech and lottery industry At Medius we are helping companies unlock their machine learning potential by finding real use cases that perform and bring added value to the whole value chain.