S strojnim učenjem do učinkovitejšega vzdrževanja vodovodnega omrežja
PipeAI - Zgodba o uspehu
Vodovodna omrežja so z vidika varnosti in funkcionalnosti ena najbolj kritičnih infrastruktur sodobne družbe. Odkrivanje izgube vode pa zaradi svoje kompleksnosti, pomanjkanja razpoložljivih podatkov in dejstva, da jih ni lahko odkriti, ostaja ena težjih nalog. Do zdaj.
Za trenutek si predstavljajmo rešitev, ki lahko samodejno predvidi izgube vode na vodovodnem omrežju in jih locira še preden se na omrežju zgodi vidna škoda. Takšna rešitev bi podjetjem, ki upravljajo z vodovodnim omrežjem, prihranila veliko denarja in virov, obenem pa bi končnim uporabnikom prinesla številne koristi.
To je bil eden naših ciljev, ko smo zasnovali rešitev z imenom PipeAI.
Kaj je PipeAI?
PipeAI je sistem, ki nam s pomočjo strojnega učenja pomaga odkrivati točke na vodovodnem omrežju, kjer prihaja do izgube vode. Gre za samonapajalni sistem za upravljanje vodnega omrežja, ki omogoča neprekinjeno spremljanje na daljavo in odkrivanje napak na cevovodu, kar počne na podlagi podatkov s terena in satelitskih podatkov. Končna rešitev pripomore k boljšemu prepoznavanju napak na vodovodnem omrežju in omogoča pravočasno odkrivanje točk, kjer bi lahko prišlo do napak. Ker sistem PipeAI poganja strojno učenje, se skozi čas stalno uči in izboljšuje.
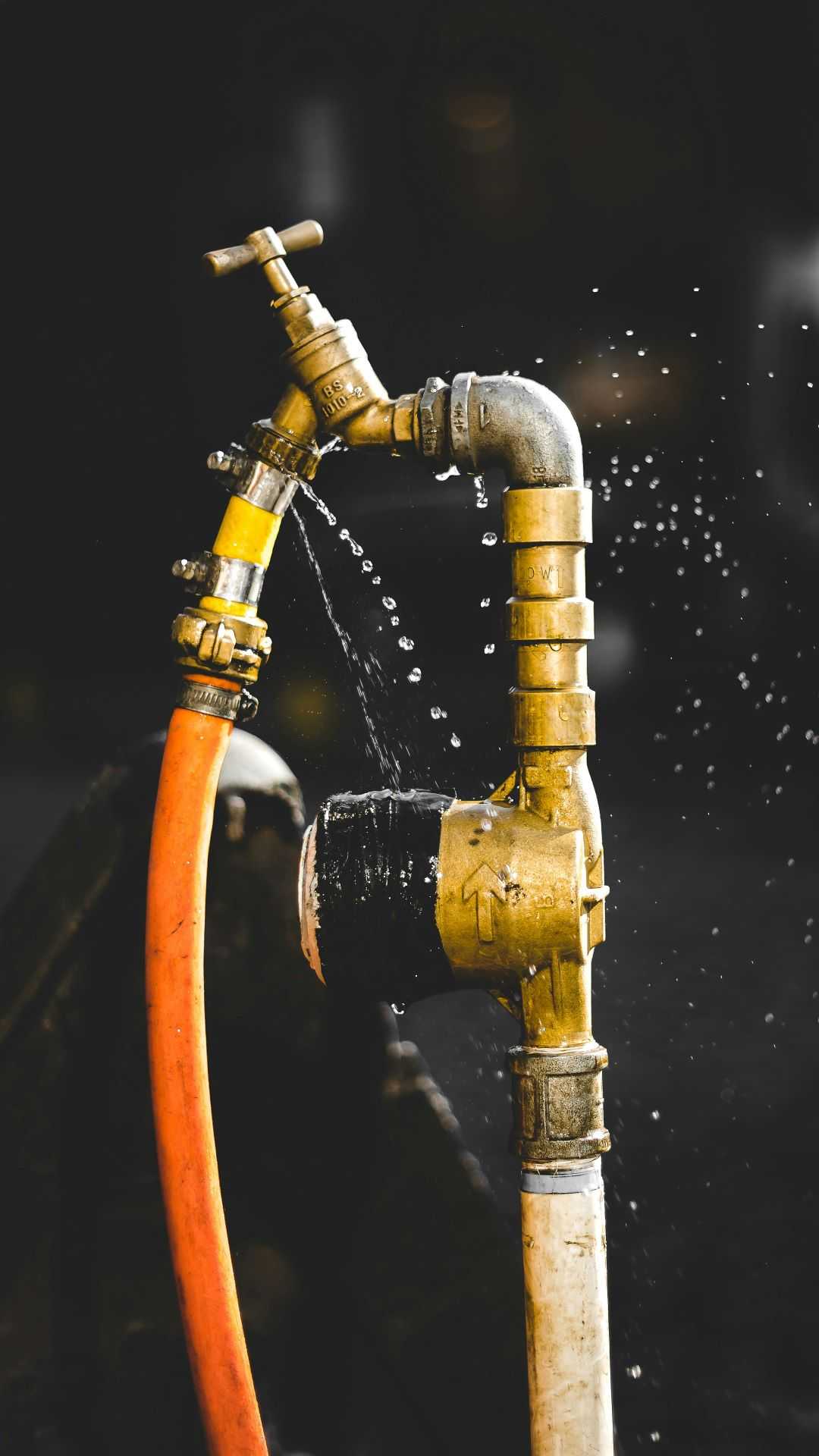
Fotografija: Valentin Petkov na Unsplashu
Uporaba PipeAI na vodovodnem omrežju zmanjšuje napake na cevovodu in povečuje prihranke vode.
Kako deluje?
Naša rešitev temelji na analizi in obdelavi podatkov z uporabo modelov strojnega učenja, ki nato vplivajo na delovanje omrežja. Dve glavni podatkovni točki, ki ju uporabljamo za ustvarjanje algoritma za napovedovanje okvar cevi in puščanja vode, sta podatki iz senzorjev na cevovodu in podatki iz satelitskih sistemov za odkrivanje puščanja.
Vendar kako satelit ve, kaj se dogaja v cevovodih? Ena od satelitskih informacij, ki jih obdeluje naš algoritem, je vegetacija ali bolje, spremembe v vegetaciji na površini trase cevoovoda. Še natančneje, sistem PipeAI opazuje količino trave ali mahu na površini in se sčasoma nauči razlikovati med normalno rastjo vegetacije in spremembami, ki bi lahko pomenile izgubo vode v sistemu. Kadar je to mogoče, s pomočjo tehnologije za obdelavo slik ovrednotimo tudi temperaturne razlike na površini cevovoda in tako zaznamo morebitne spremembe na sistemu. Se sliši neverjetno? Ampak ni.
Z uporabo sistema PipeAI in strojnega učenja v realnem času lahko pri spremljanju stanja na cevovodu dosežemo neprimerljiv nivo zanesljivosti, kar pomeni manj okvar na cevovodih in večje prihranke vode. Koristi za lokalne skupnosti, okolje in predvsem podjetja, ki upravljajo s cevovodi, so izjemne. Na tem mestu je nujno omeniti še vso prihranjeno energijo, nižjo potrebo po popravilih in s tem nižje stroške vzdrževanja cevovoda.
In prav zato je primer PipeAI še ena zgodba o uspehu, ki smo jo preprosto morali deliti z vami.